How we are using A-B testing to refine our pricing model – Part 1
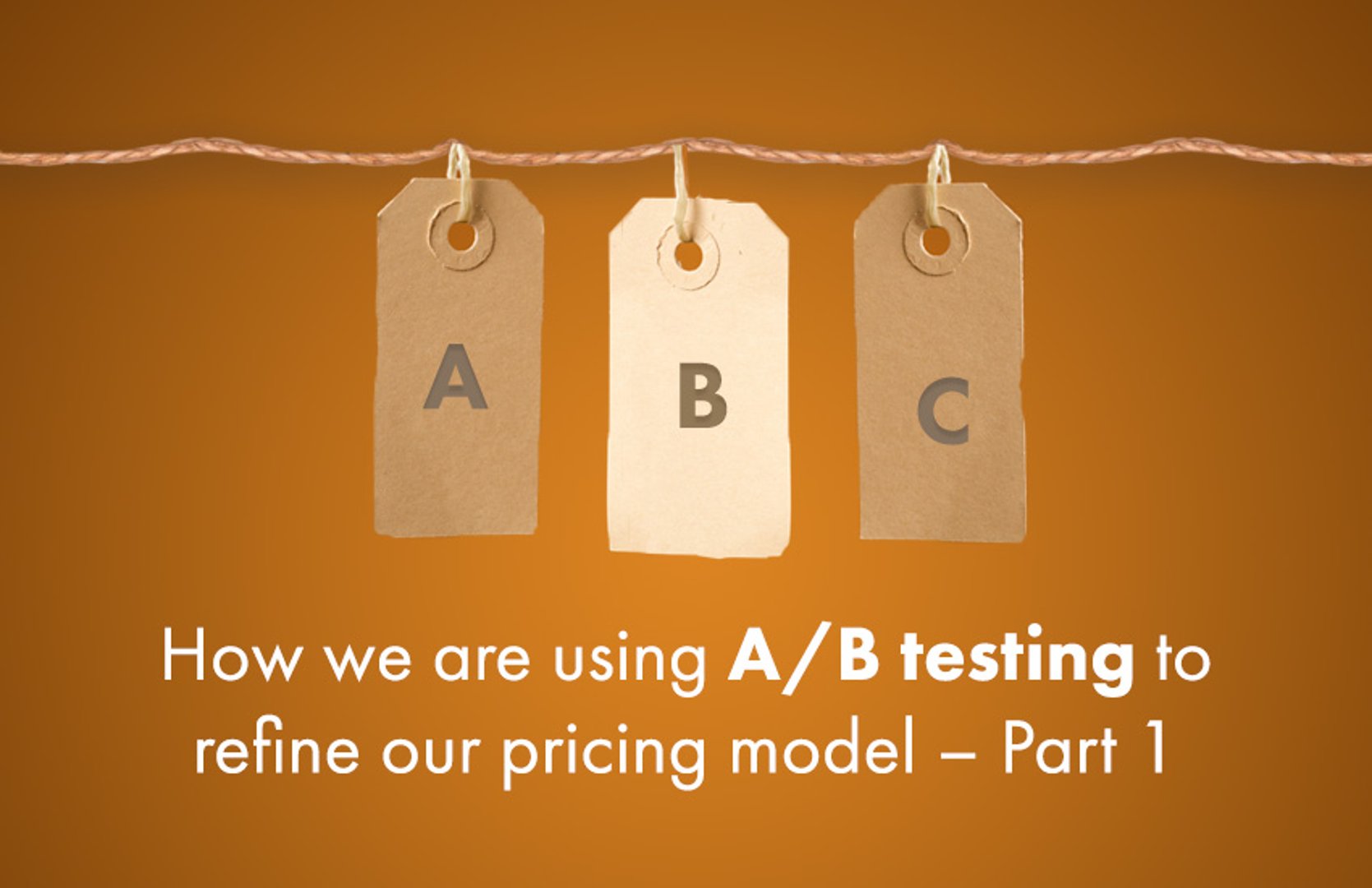
Published 2023-03-21
Summary - In 2012, Klipfolio created a pricing model based on the type of customer we were looking to attract at the time. But we’ve recently refocused our objectives, and have decided to target small and medium-sized businesses (SMBs) instead of somewhat larger companies. We suspected that our pricing model was not suited to our new target audience, and worked to put together the
Pricing is hard to get right. It’s not well understood, and it’s rarely optimized.
Setting a price isn’t about mimicking your competitors. It’s about setting a price – and possibly a bundle or package – that’s both right for your target market and aligned with your goals.
For example, would people buy more eggs if they were sold individually instead of by the dozen? How about if eggs were sold in a breakfast bundle that included bacon, coffee, toast and orange juice, all in one package? Would that work?
Take the time to understand what pricing model works best for you. Most people don’t. I’d say that most companies merely copy their competitors, and for lack of a scientific process, just guess. I feel strongly that a business needs to actively test various models to make sure the price it sets is strategic and aligns with its objectives.
This week, I want to talk about how we created a system to test two very different pricing models for Klipfolio, and why.
Next week, I’ll talk about the results.
Let’s start with the testing.
In 2012, Klipfolio implemented a pricing model based on the number of users within a customer account.
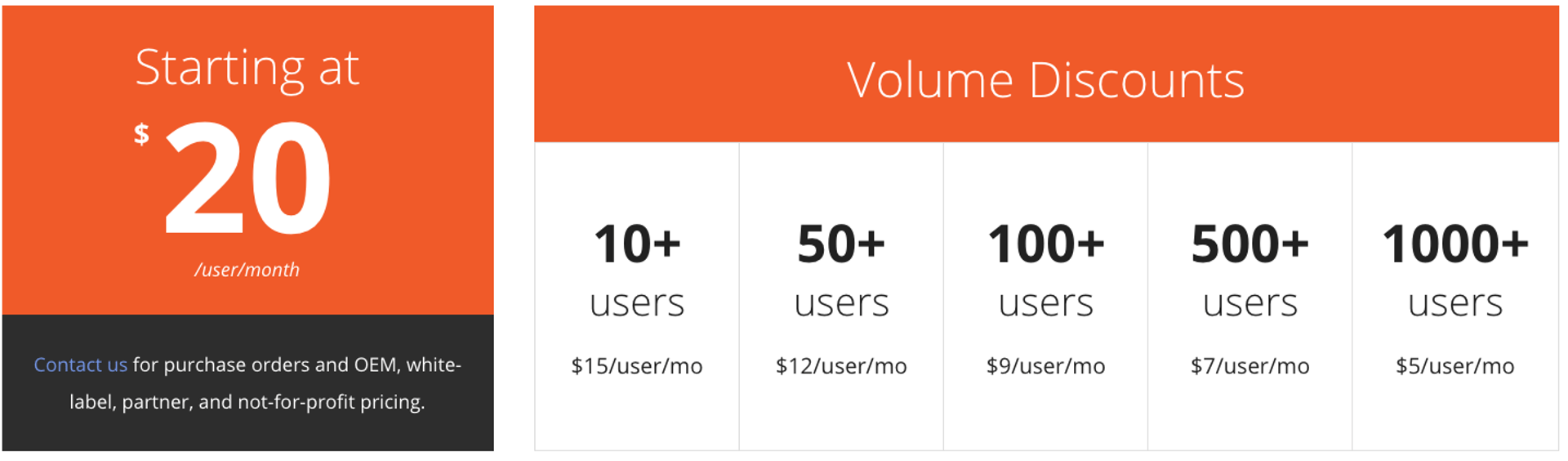
It’s pretty straightforward, and likely the most common pricing model out there.
A customer is charged based on the number of registered users it has. There is no limit on what each registered user can do – each has access to every single feature we create. Customers do, however, get a break on volume: We offer discounts to customers with a large number of users.
But here’s the rub: Market share and massive user adoption are really key to our growth strategy. And how can we maximize that when our pricing model is actually an inhibitor to user growth?
Was our 2012 model aligned with our strategic objectives?
The only way to find out was to do a test.
A-B testing is a very simple concept: You divide your audience and present each group with a different version of something to see which does better.
For example, a company might want to see which of two web page designs results in more sales by directing some site visitors to one version, and other site visitors to another.
When you compare results, you see which design works best.
We decided we would do an A-B test of two pricing models: the existing one, and a new one based not on the number of users a customer has, but on the number of resources and features the customer uses. I’ll share a screenshot of the new pricing model next week.
The premise was that by allowing more users, the value of our tool would be more apparent: More users in an account would mean more opportunity for success because users can collaborate, build more, share more and generally get a better sense of the value of the tool.
In fact, we suspected that limiting the number of users of an account was the equivalent to keeping one foot on the brake when it came to growth. Our existing pricing model was (we hypothesized) actually impeding our growth by limiting our attractiveness to SMBs.
We’d done A-B testing before, but this was going to be the biggest and most important A-B test in our company’s history.
First, it was important that people not know they were being tested. We needed to create a system that would direct some new customers to the existing pricing system, and some to the new one, and we had to make the experience seamless, from first visit to our website through to their trial of our dashboard. If a visitor to our website was directed to Option A once, he or she had to be directed to Option A every time they clicked onto our site or tried our dashboard.
Although we use Optimizely extensively for most of our A-B tests, this time we built our own logic to make this happen.
Second, we created a testing framework we could use over and over again. Everyone we talked to advised us that the only true way to optimize your pricing model is to test it - and to do it several times a year. So we put a lot of effort into building a reusable testing framework.
And third, we had to get over our fears about what the whole testing experience would do to our existing and potential clients.
For example, what if people found out we were testing two pricing options?
We decided we’d be upfront and tell them - and learn in the process which model they’d prefer.
In late November, once everything was place, we quietly began our A-B test on pricing.
The test ran for more than two months. Next week, I’ll talk about the results.
Allan Wille is a Co-Founder and Chief Innovation Officer of Klipfolio. He’s also a designer, a cyclist, a father and a resolute optimist.
Related Articles
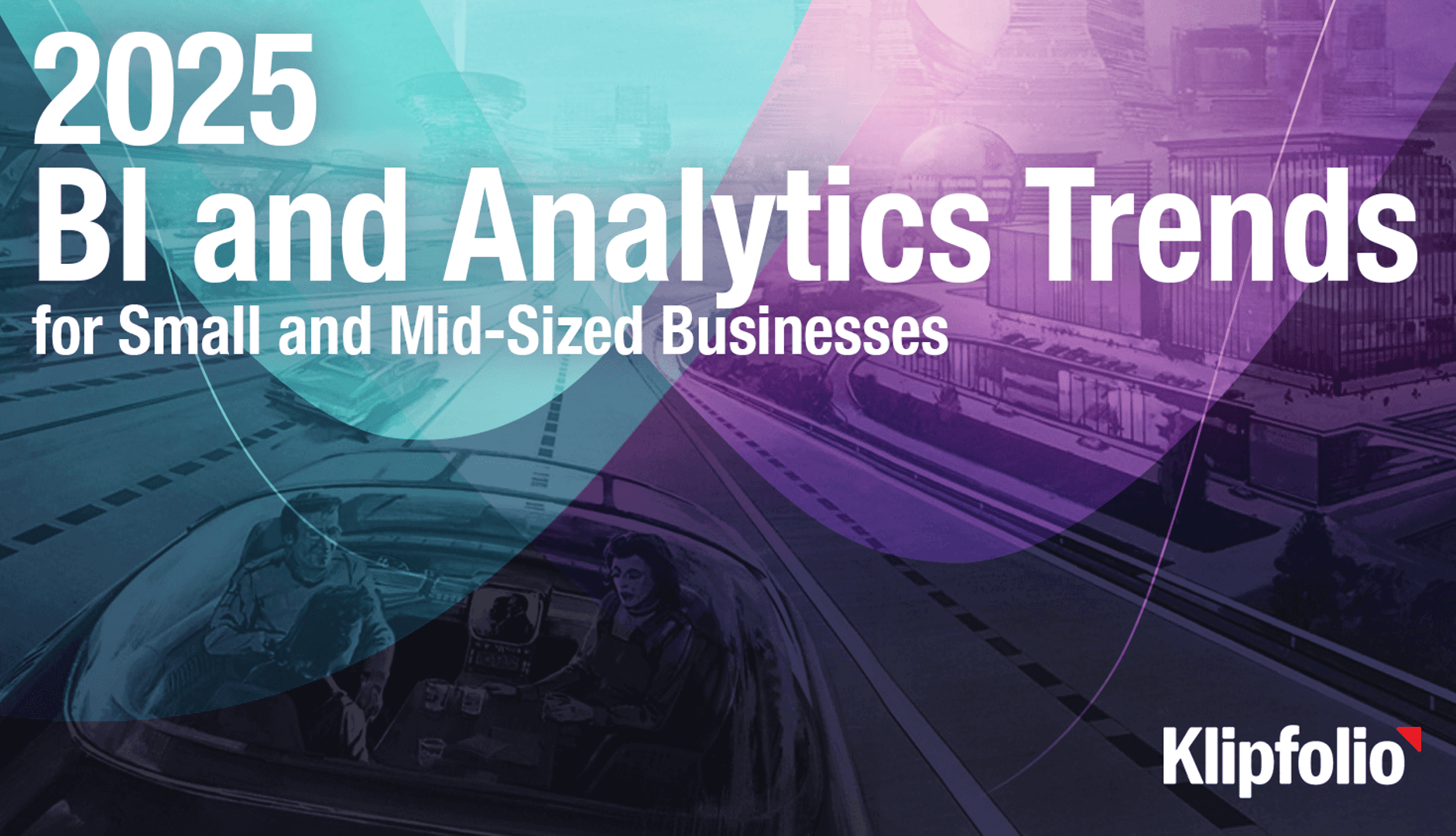
2025 BI and Analytics Trends for Small and Mid-Sized Businesses
By Allan Wille, Co-Founder — December 18th, 2024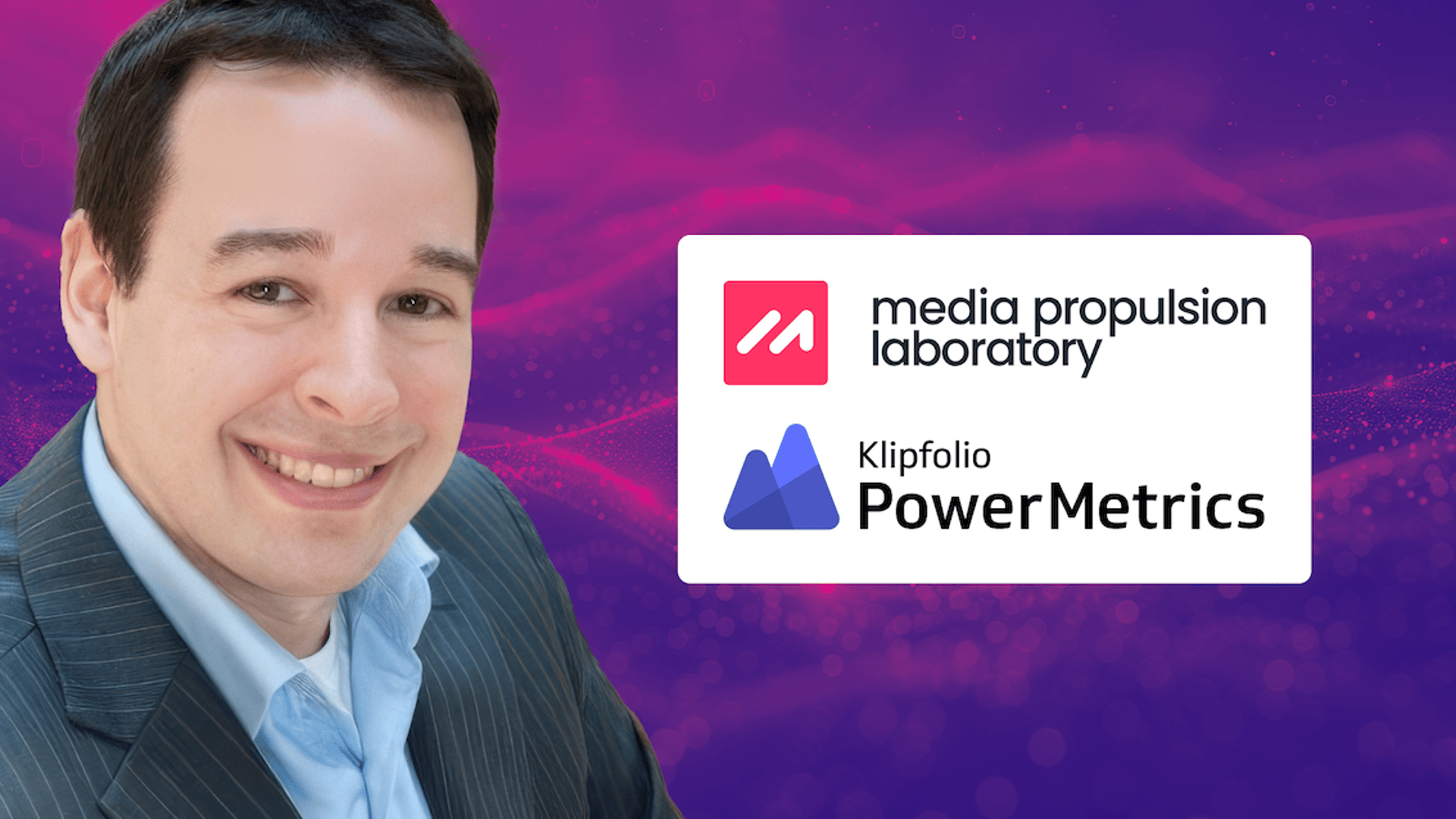
Case Study: How Media Propulsion Laboratory improved client analytics with the PowerMetrics – BigQuery integration
By Cathrin Schneider — September 12th, 2024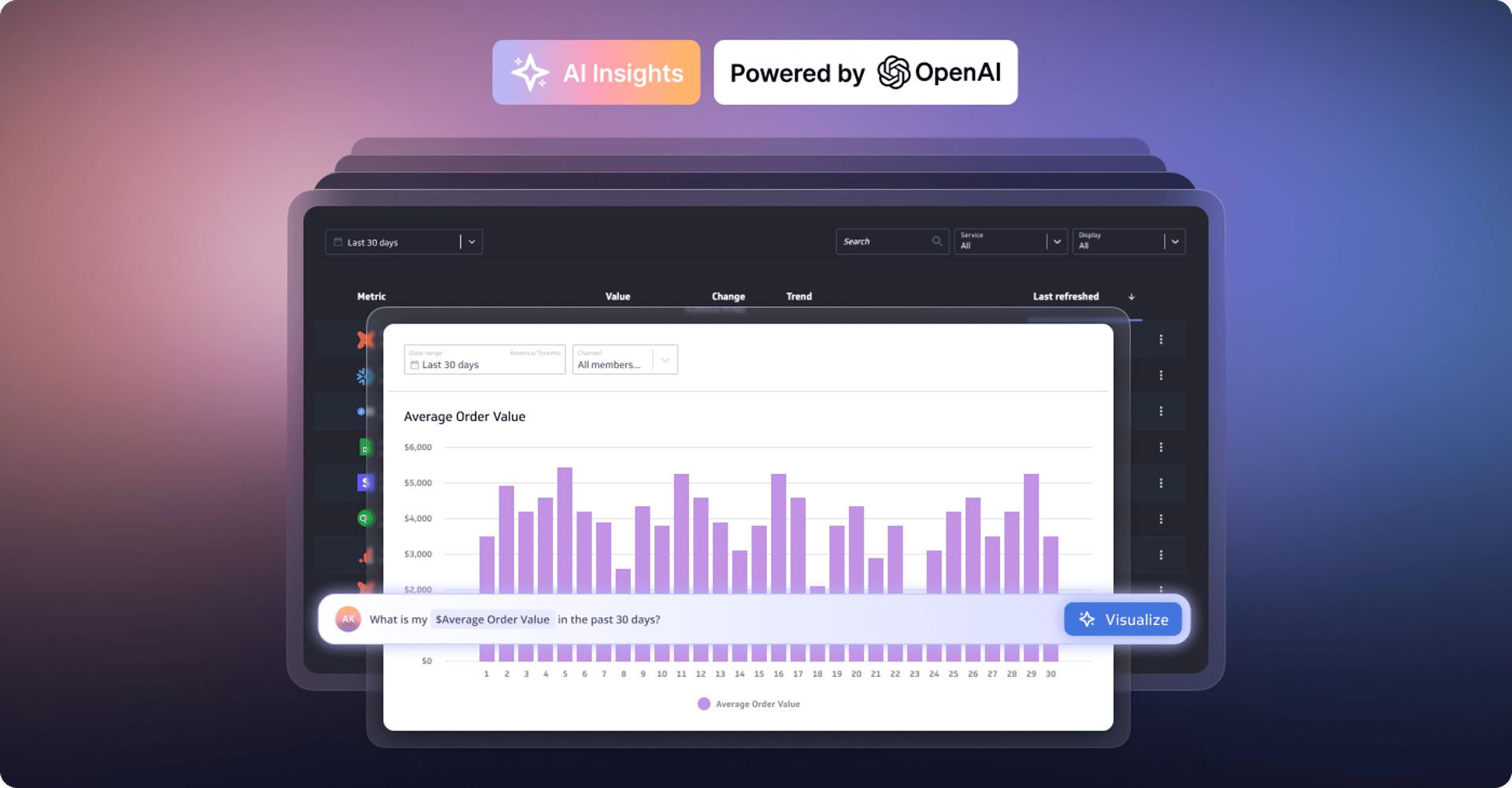
Metrics provide rich context for better AI: Introducing the new PowerMetrics AI experience
By David Mennie — June 21st, 2024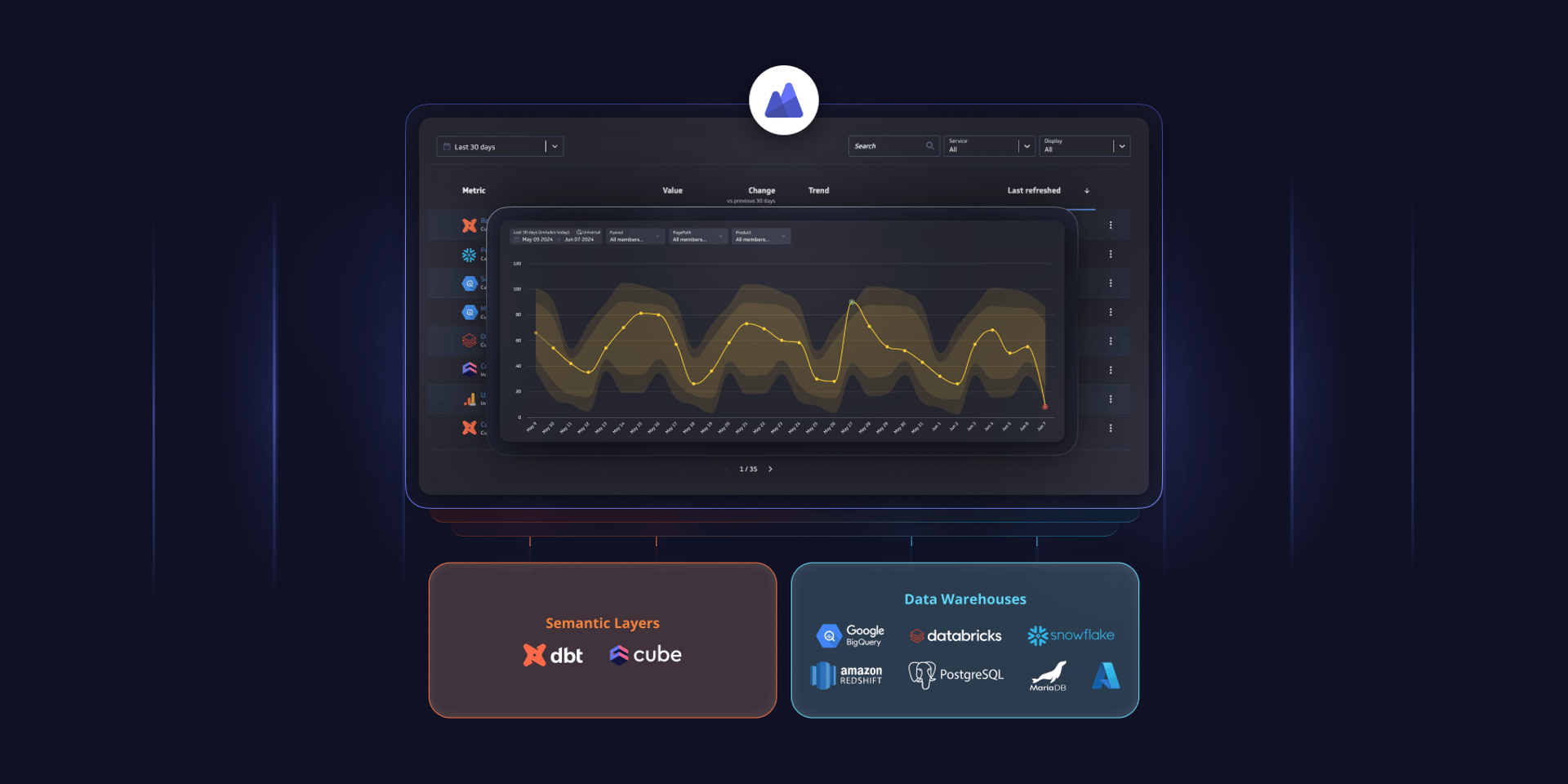